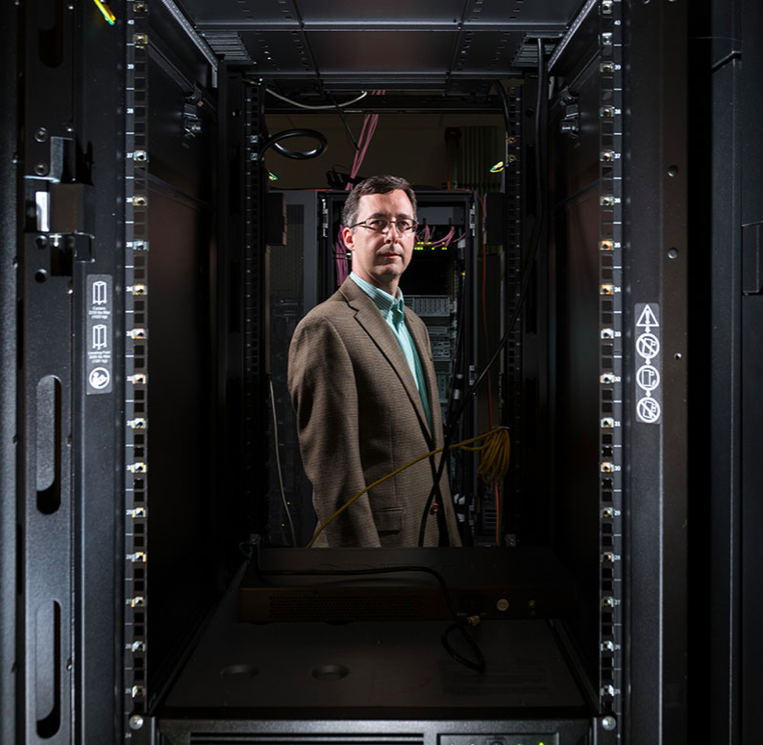
Mar 30, 2020 - Atlanta, GA
Scientists know exactly where to look for potential weaknesses in viruses that cause disease: their protein shells.
The genetic material inside a virus is surrounded by a protein-based covering called a capsid. Searches for therapeutic treatments and vaccines are now focusing on proteins like capsids that drugs could attack, and a highly-read study from a Georgia Tech research group is offering expertise to aid in efforts like these.
The Sherrill Group is studying artificial intelligence and machine learning in the hopes of making drug discovery faster and more efficient, says David Sherrill, a computational chemist and professor in the School of Chemistry and Biochemistry and School of Computational Science and Engineering, who also serves as associate director of the Georgia Tech Institute for Data Engineering and Science. “AI promises to help us identify druggable target proteins, to predict what drugs might be effective, and even how to synthesize those drugs.”
The team has created the first pure machine learning model designed specifically for intermolecular interactions, such as those governing a drug binding to its target proteins.
The models described in a study published recently in The Journal of Chemical Physics. Sherrill and Derek Metcalf, a Ph.D. student in his group, have also given recent lectures on their research.
Tiny Molecules Meet Big Data
Sherrill says much work has already been done in chemistry research using machine learning, where computers search for patterns and possible connections within data on their own without specific instructions. But so far that approach “has gone into predicting properties of single small molecules. Intermolecular interactions pose several subtle problems that we elucidate” in the study, he says.
The drug design process can benefit from artificial intelligence in several ways, including more accurate estimates of how drugs move within the body, and better predictions about their synthesizability.
In the initial pilot study, the researchers developed a way to encode high-level quantum (subatomic) mechanical computations on molecule-to-molecule interactions into a machine learning model. “Tests indicate that the model is promising, and it is much faster than the corresponding quantum computations, which involve fractions of a second for machine learning, compared to hours for quantum mechanics,” Sherrill says.
“Although our initial study was focused primarily on hydrogen bonds, the group has already developed a more general model applicable to all the kinds of molecule-molecule interactions involved in drug-protein binding, and we are currently in the process of generating the large data set of quantum data required to train the model,” he says.
Ultimately, the team hopes to develop a model that will be nearly as accurate as quantum mechanics, while making predictions almost instantaneously. Such a model could be very helpful in screening extremely large numbers of possible drug molecules for their ability to bind to a protein.
During his time at Georgia Tech, Sherrill’s work has focused on the intersection of computer software, chemistry, and physics. He is one of the co-principal investigators for the Georgia Tech Supercomputer Hive.
Sherrill also leads the Georgia Tech team that wrote Psi4, a suite of open-source quantum chemistry programs that Google selected in 2017 as a plug-in for OpenFermion, its free and open-source chemistry package for quantum computers.
He is a Fellow of the American Association for the Advancement of Science (AAAS), the American Chemical Society, and the American Physical Society, and he has been Associate Editor of the Journal of Chemical Physics since 2009.
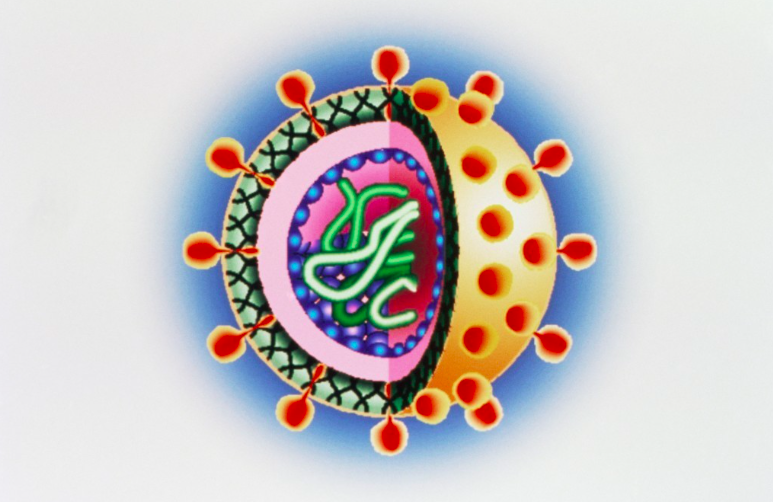